Scientists use uncrewed surface vehicle to estimate krill abundance in Alaska
by NOAA Fisheries 22 Feb 16:58 UTC
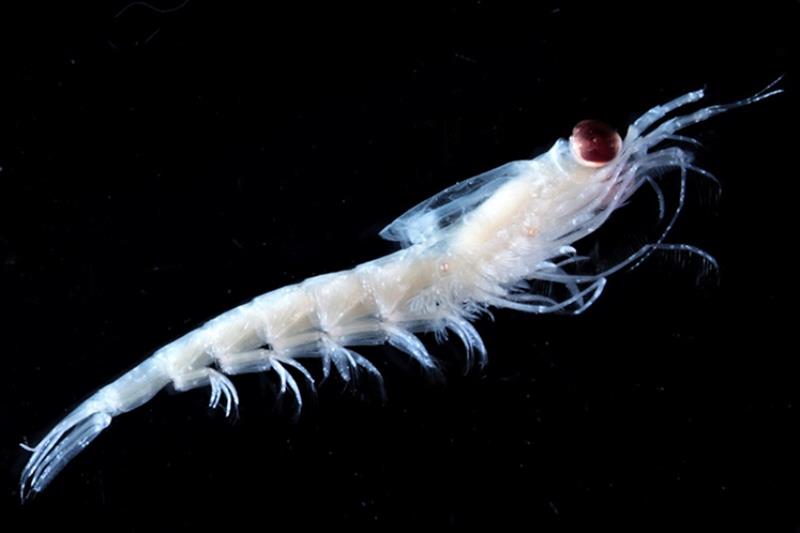
Krill (Thysanoessa inermis) © NOAA Fisheries
Throughout the world, scientists engage in regular vessel surveys to produce estimates of species distribution and abundance. These estimates let scientists track species- and ecosystem-level trends over time to support ecosystem-based fishery management.
Increasingly, scientists are exploring ways to conduct these important surveys more efficiently without jeopardizing the quality of the data they have collected consistently.
In a new study, NOAA Fisheries scientists filled a data gap by combining observations from uncrewed surface vehicles and a commonly used machine-learning algorithm. As a result, they were able to estimate krill abundance in 2020 when a standard vessel survey was not possible.
In the eastern Bering Sea, scientists conduct biennial acoustic-trawl surveys from the NOAA Ship Oscar Dyson, a crewed vessel. The primary goal of these surveys is to estimate walleye pollock abundance and distribution. This commercially valuable fishery is the largest volume fishery in the United States. However, the acoustic-trawl surveys have also provided estimates of krill abundance extending back to 2004.
Krill are a major component of the eastern Bering Sea ecosystem, providing an important food source for fish, seabirds, and marine mammals. Thus, krill abundance is an important indicator of ecosystem status and prey availability. In 2020, the Covid pandemic forced the cancellation of the eastern Bering Sea acoustic-trawl survey.
A combination of technological approaches supports long-term science
As a partial replacement for the lost survey effort in 2020, scientists used three saildrones to collect acoustic data. They still were able to estimate pollock abundance and distribution despite the survey cancellation. In this study, these same data also were used to estimate krill abundance and distribution.
"The big challenge we faced using uncrewed surface vehicles to estimate krill abundance was that we had less informative acoustic data available than we did from our NOAA research vessel," said Mike Levine, lead author of the study and fisheries biologist at the Alaska Fisheries Science Center. "The sonar instruments these uncrewed systems carried had to be more compact and only provided acoustic data at two frequencies. This contrasts with the standard vessel-based survey, which provides four-frequency acoustic data for krill estimates."
Scientists faced a challenge. Four frequencies have traditionally been used in this survey to identify krill. Applying the standard processing algorithm with two frequencies instead of four produced an estimate that was much more uncertain and overestimated krill abundance.
The solution was to apply a simple machine learning approach known as a random forest algorithm. Scientists trained the model to identify krill with only two frequencies using data from previous crewed surveys. They checked the model performance by running the method on previous surveys not included in the training data, then compared the results to the standard four-frequency result.
The random forest approach worked. It produced a comparable estimate to the standard survey for the year of missing data. Scientists were able to keep the time series going despite the lack of a ship-based survey in 2020. Still, the results of the two-frequency random forest approach are more uncertain than those based on four frequencies. Additionally, there is a loss of trawl sampling for krill, which allows scientists to collect other important biological information (e.g., species and size).
"In many situations, the loss of trawl sampling is a major limitation in the use of uncrewed vehicles in place of ship-based abundance surveys. The uncrewed surface vehicle approach worked well to 'fill in' 1 year of missing krill survey data in this case because we use a multi-year average of size and species composition to estimate krill in the eastern Bering Sea," said Alex De Robertis, coauthor and fisheries biologist from Alaska Fisheries Science Center. "However, we really need to be able to do direct sampling or we could miss long-term changes in krill size, species composition, or range. Thus, continued trawl validation of acoustic data is prudent."
Large-scale surveys can be disrupted for any number of reasons—such as mechanical breakdown, instrument failure, and even unexpected events like a global pandemic. Uncrewed surface vehicles provide a pragmatic way to address some of the challenges faced in continuing long-term survey time series. However, uncrewed surface vehicles may provide scientists with less or different information than survey vessels. This study provides an example of how machine learning can be used to maximize the information derived from these data sources.
Scientists found that the random forest approach can be a simple, straightforward method to implement, and an effective tool to address changes in survey methods or data.